Inside the Wise Leader’s Brain 4 | Meet your Statistical Brain Team
Scientists formulate hypotheses and apply them to probabilities and uncertainties. So does our brain. It selects the hypotheses that best fit with our environment. As it hypothesizes, as it collects data and re-adjusts, it learns.
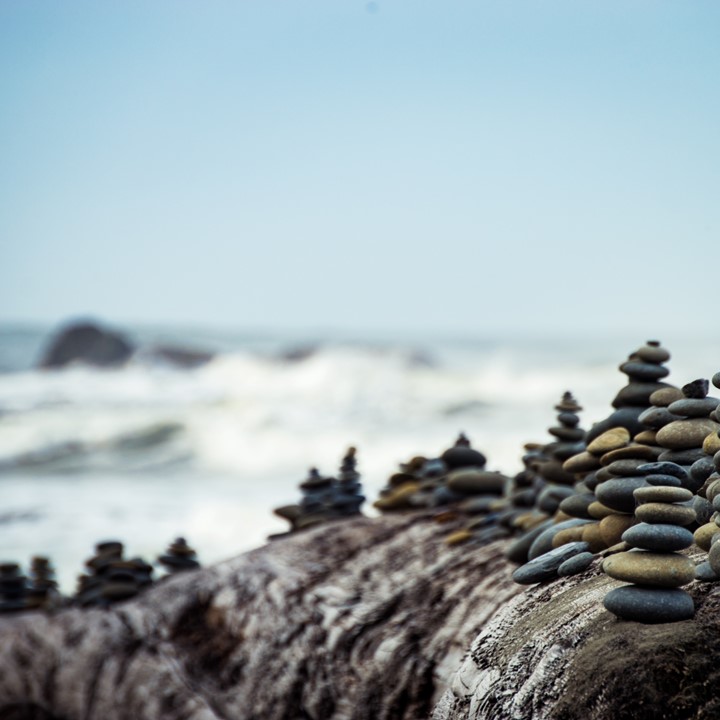
It's probably a cat: evolution wired our brain for prediction
Millions of nonstop predictions give meaning to the external environment that our senses perceive, and so our brain constructs the world we experience. During its evolution, our brain seems to have acquired these sophisticated algorithms that constantly keep track of the uncertainty associated with what it has learnt.
Deeply inscribed in the logic of our learning, reasoning with probabilities happens under the radar. A whole array of mental processes can be launched without consciousness; even though, in most cases, they don’t run to full completion.
Conscious perception has a major role to play, too. It transforms incoming information into an internal code that allows it to be processed in unique ways. Our brain uses a division of labor: an army of unconscious statisticians and a single decision maker (or “interpreter”).
A strict logic governs the brain’s unconscious circuits
These appear ideally organized to perform statistically-accurate inferences from sensory inputs. Our unconscious perception works out the probabilities, our consciousness takes a random sample. Consciousness acts as a discrete measurement device that gives us a glimpse of the vast underlying sea of unconscious computations. The mighty unconscious generates sophisticated hunches, but only a conscious mind can rationally think through a problem. Language (and memory), put together, allow us to structure our mental world and share it with other minds.
All (human) knowledge is based on two components
Firstly, a set of innate ‘a priori’ assumptions, before any interaction with the environment, and secondly, the capacity to sort them out according to an ‘a posteriori’ plausibility, once we have met some real data.
Adjusting as we go
Through prediction and correction, the human brain continually creates and revises our mental model of the world. This huge, ongoing simulation constructs everything we perceive, while determining how to act. Even babies seem to understand probabilities, deeply embedded in their brain circuits (“if I cry, I’ll get some attention around here...”).
Humans are very good at making decisions with limited data, but not when overloaded. Machine learning algorithms, on the other hand, are very good at finding patterns in big data.
In machines, just as in humans, learning always starts from a set of hypotheses. These are projected onto the incoming data and the system selects the ones that best fit the current context. Despite this similarity, our brain (so far) learns better than machines do.
The idea that every unexpected event leads to an adjustment of the internal model of the world is also known as the ‘theory of error backpropagation’ — we visited error backpropagation earlier when discussing supervised machine learning. Learning by error correction is a feature not only of AI and humans, but animals too.
Returning to our pyramid image, the brain is a massive hierarchy of predictive systems. Generating a prediction, detecting our error, and correcting ourselves, are the foundations of effective learning. Stanford psychologist Carol Dweck has called this a growth mindset, versus a fixed mindset.
Once again, the result is a compromise, a selection, of the best internal model from the selection our prior organization makes available to us. A (young unexperienced) person ‘knows’ what a face (a conceptual output) looks like. A human can easily recognize and thus predict a specific face. A computer doesn’t have this pre-understanding. Through layering and error backpropagation (which we can also see as feedback), the input of an “object face” finally enables it to see “the face of x”. Moreover, a human can be self-confident and show confidence in others.
Going Deeper | Footnotes
On models: Humans use models to understand. Indeed, we create models of the future by recruiting our memories of the past. We draw from the past in order to imagine what lies ahead. Mentally, we are all time travelers. And what gives life meaning is the rich and constant interplay between past, present and future. Our brains are “prediction machines” helping us to see the future, to prepare us to find meaning in all the phenomena (or data input) we encounter. Memory requires a state of alertness, attentiveness, and awareness of the present. At the same time, our capacity to generate a vast range of scenarios is what gives us the capacity for change. Humans map thousands of possibilities with a lively, free-flowing combination of routine and creative prescription and foresight knowledge. (Heffernan, 2020).
On predictions: “Your brain’s colossal, ongoing storm of predictions and corrections can be thought of as billions of tiny droplets. Each little droplet represents a certain wiring arrangement – a prediction loop… Neurons participate in prediction loops with other neurons…[…] As each prediction propagates through millions of prediction loops, your brain simulates the sights, sounds, and other sensations that the predictions represent, as well as the actions you will take…[…] Your brain works like a scientist. It’s always making a slew of predictions, just as a scientist makes competing hypotheses. […] Your brain does not just predict the future; it can imagine the future as well. As far as we know, no other animal brain can do that.” (Lisa Barrett, 2017).
In the next chapter, we’ll explore the problem with homo deus.
By Dr. Peter Verhezen, with the Amrop Editorial Board
Peter is Visiting Professor for Business in Emerging Markets and Strategy and Sustainability at the University of Antwerp and Antwerp Management School (Belgium). He is Principal of Verhezen & Associates, and Senior Consultant in Governance at the International Finance Corporation (World Bank) in Asia Pacific. In this capacity, he advises boards and top executives on governance, risk management and responsible leadership. Peter has authored a number of articles and books in the domain, and collaborated closely with Amrop in the development of the wise leadership concept.